Sentiment Analysis, also known as Opinion Mining, is an example of data mining, which means to explore the preference or tendency of people about varied topics. With the explosion of data spreading over various web social media, like Twitter and more, many people have the needs of crawling the websites. Thus, data mining has also been widely used in different industries for different uses.
To start with Sentiment Analysis, what comes first is to crawl data with high quality. Normally, people crawl the web to access the data resource. There are several ways for us to crawl the websites. Some websites have provided public APIs for people to crawl their data set, like Facebook, Twitter. However, if people would like to crawl more data not available in their public data set, people have to build a crawler on their own by programming or using certain automated web crawler tool like Octoparse.
We encourage people to choose crawling websites by programming using Ruby or Python. But people without any coding skills or who want to save more time can choose a certain professional automated crawler tool, like Octoparse based on my user experience.
Data Crawling Tool
We now need to conduct an experiment on “Bag-of-words”. To start with our analysis, we should collect data first. As mentioned before, there are several methods we can use to crawl website data. In this writing, I’d like to propose an automated web crawler tool – Octoparse for your convenience to crawl the data you need.
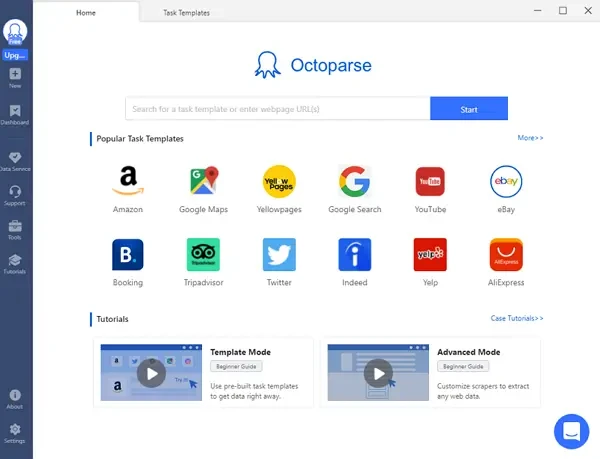
Octoparse is a powerful data collection tool that can help you to collect/crawl millions of data from most websites. It’s designed for non-programmers since users can just get customized crawled data results by simply pointing & clicking on a user-friendly interactive interface which is called “Custom Task”. Users can reformat the data and locate the exact way of particular irregular data fields manually. Moreover, Octoparse provides more than 50 pre-built templates. For the pre-built templates, the template scrapers will do the job for you as long as filling in the required parameters instead of building your own scraper to get what you want. Octoparse has many powerful and useful features, such as proxy service, cloud service, solving captcha and more. You can use it to scrape different types of websites, like Amazon, eBay, AliExpress, Priceline and others including price, reviews, and comments data.
If you have the needs of web scraping and are wondering which tool can assist you to scrape quickly and easily. Octoparse is recommended. You will be surprised by its powerful features, especially its updates with the new version 8.5.4 recently. No more waiting, it can reduce your time cost greatly for getting the data you want, even though it is a large amount of data. Download and play around with it now.
After completion of the scraping task either by programming or using scraper tool like Octoparse. We can randomly select 8 books with their respective customer reviews.
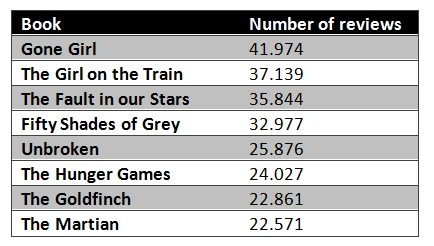
Then we can score these selected books and visualize these scores using a column graph as the graph shown below. As we can see, the distribution and trend of the score are reasonable and characteristic since very few star ‘1’ is left in the comments board of books with over-averaged score, while the score shows certain specific trend for those books with below-averaged score. Here, by observing the data we crawled, we can derive that Gone Girl suits well for our training data set, while Unbroken doesn’t fit our training set since there is almost none ‘1’ star comment with it.
Python: “Bag-of-words” Model
“Bag-of-words” Model has been working well with subject classification, while not that accurate when analyzing Sentiment Analysis. One Sentiment Analysis research on movie reviews conducted by Bo Pang and Lilian Lee in 2002 shows an accuracy of mere 69%. However, if we use Naive Bayes、Maximum Entropy、Support Vector Machines which are common text classifier, then we can get a higher accuracy around 80%.
While, the reason we choose “Bag-of-words” Model is for the reason it can help us to deep learn the text content, and the three commonly used classifers are also based on “Bag-of-words” Model which could be deemed as an intermediate method.
So far, the NLP(Natural Language Processing)has been committing itself in dealing with the bag-of-word. Most of its work is applied in the field of machine learning based on statistics. Some people may be not acquainted with the conception of Bag-of-word. So, what Bag-of-word really means? As known, the object of NLP is the natural language text. Specifically, comments, reviews, corpus, document, post, text with discourse all can be the input of the system of NLP. After the input of NLP, what comes next is tokenization. To put it another way, a bag-of-word is to tokenize the input natural language, and process with those tokenizations based on statistic models.
After completion of crawling web data, we categorize the comments data we crawled before into “Training Data Set” and “Testing Data Set”. There are around 40000 comments fo r “Gone Girl” in total. And we decide to use half of the data as our “Training Data Set”, and the rest of crawled data will be used for “Testing Data Set”. Plus, to make our experiment more accurate, we will adjust the size of training data set from 1000 to 20000 comments considering the factor of training data set size.
“Bag-of-words” keeps track of the occurrence number of each word to build up the text Unigram Model which will be used as the text classifier feature then. In this model, you can only analyze the words separately and assign subjective score to them respectively. If the sum of score is lower than the standard line, we can derive this text is negative, otherwise it is positive. “Bag-of-words” sounds easy may be, however, it is not that accurate since it doesn’t consider the grammar or sequence those words. To improve it, we can combine Unigram Model with Bigram Model, which means we decide not to tokenize words which are followed by “not”, “no”, “very”, “just”, etc. The pseudocode to build a “Bag-of-words” is as below.
list_BOW = []
For each review in the training set:
Strip the newline character "\n" at the end of each review.
Place a space before and after each of the following characters: .,()[]:;"
(This prevents sentences like "I like this book.It is engaging" being interpreted as
["I", "like", "this", "book.It", "is", "engaging"].)
Tokenize the text by splitting it on spaces.
Remove tokens which consist of only a space, empty string or punctuation marks.
Append the tokens to list_BOW.
list_BOW now contains all words occurring in the training set.
Place list_BOW in a Python Counter element.
This counter now contains all occurring words together with their frequencies.
Its entries can be sorted with the most_common() method.
Generation of Sentiment Words
Here comes the issue that how we can relate the sentiment score with the whole text sentiment score. Here, we propose the method that using statistic standard derived from training data set to give the subject score of each word. Thus, we need to judge the occurence number in certain class of each word, and this can be realized by using and Dataframeaas datacontainer (Dictionary is the only way or other data formats). Code is as below.
from sets import Set
import pandas as pd
BOW_df = pd.DataFrame(0, columns=scores, index='')
words_set = Set()
for review in training_set:
score = review['score']
text = review['review_text']
splitted_text = split_text(text)
for word in splitted_text:
if word not in words_set:
words_set.add(word)
BOW_df.loc[word] = [0,0,0,0,0]
BOW_df.ix[word][score] += 1
else:
BOW_df.ix[word][score] += 1
def expand_around_chars(text, characters):
for char in characters:
text = text.replace(char, " "+char+" "1
return text
def split_text(text):
text = strip_quotations_newline(text)
text = expand_around_chars(text, '".,()[]{}:;')
splitted_text = text.split(" ")
cleaned_text = [x for x in splitted_text if len(x)>1]
text_lowercase = [x.lower() for x in cleaned_text]
return text_lowercase
The output result as below contains the occurrence number of each word belong to each class.
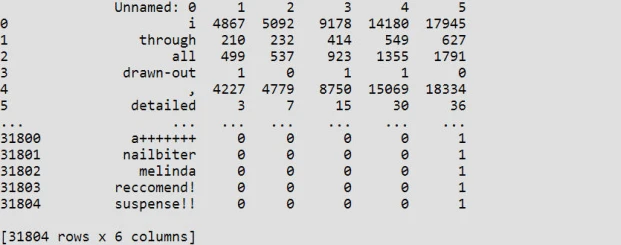
By dividing the sum number of all words which have occurred using the occurrence number of each word. We can get a relative occurrence number of each word within each class. Therefore, we can build sentiment words within this training data set and use this to evaluate the comments in the Testing Data Set.
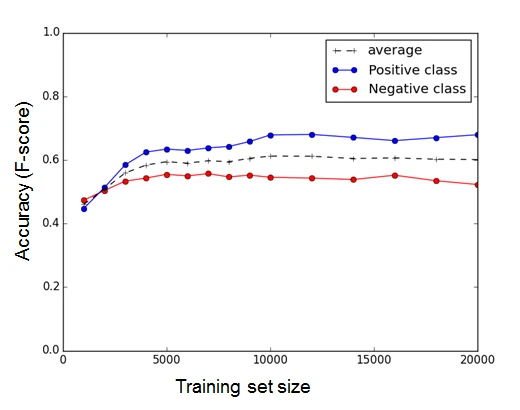
Data Evaluation
We can use “Bag-of-word” Model to evaluate whether a comment is negative or positive with an accuracy above 60% by considering ‘4’ or’ 5’ star as positive and ‘1’ or ‘2’ star as negative which you can see as the table below.
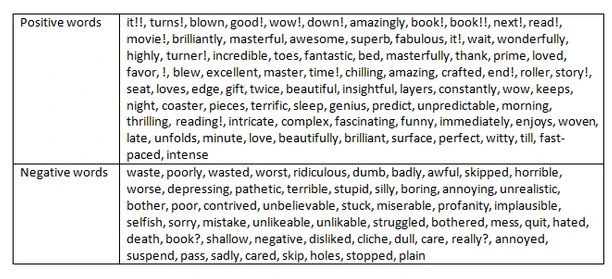