You’ve likely heard about Machine Learning many times from news and social media, but do you truly understand it? Some concepts can be challenging to grasp. Even with more learning resources like w3schools or Google being made consumable for the average reader, it still takes days or weeks for a newbie to understand.
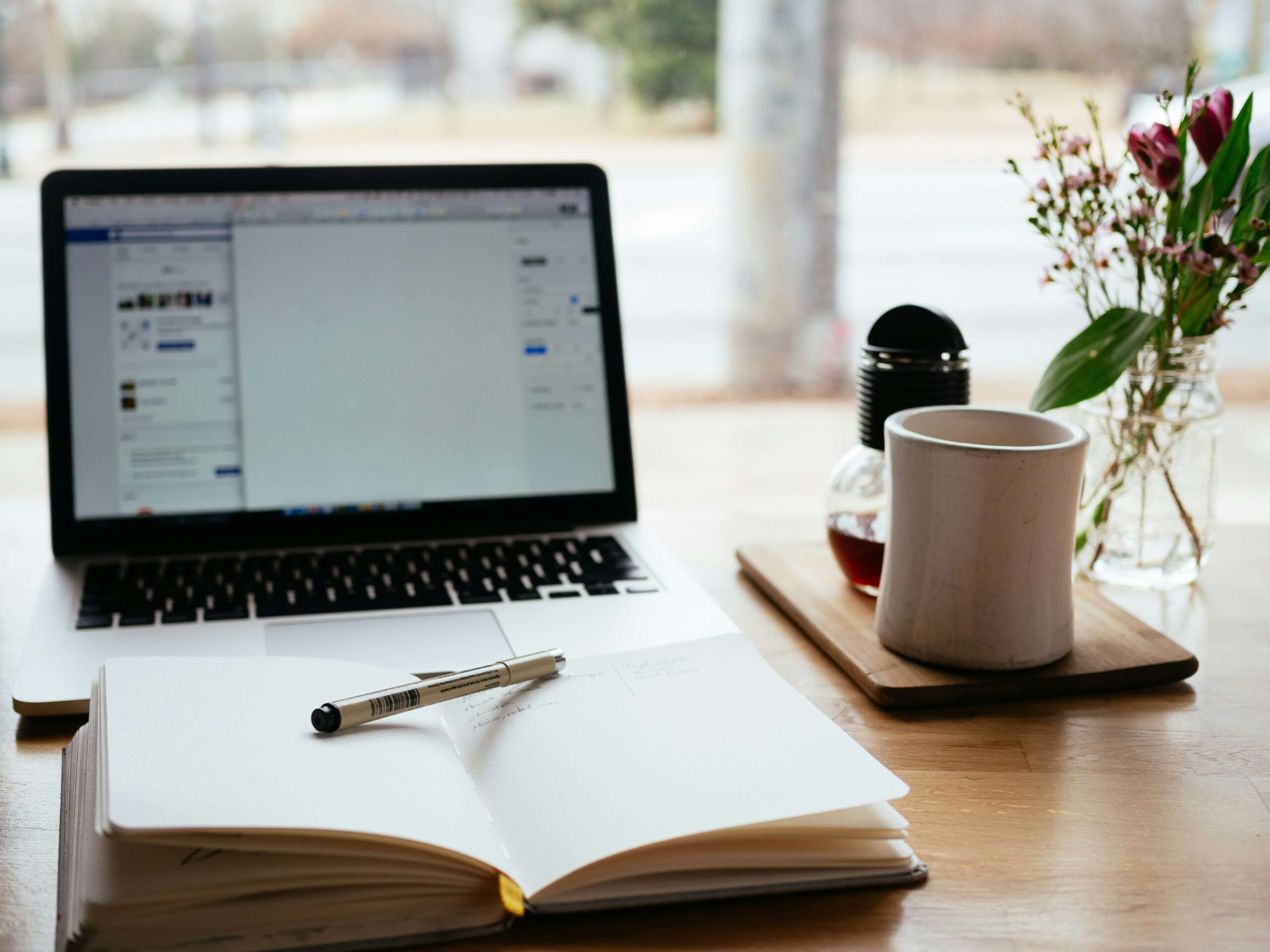
So, if you are new to machine learning, take a few minutes to familiarize yourself with the basics.You might be surprised to learn that many of the seemingly intimidating terms are quite straightforward. Without further ado, here are eight essential Machine Learning terms explained in simple words. Enjoy this post!
1. Natural language processing (NLP)
Natural Language Processing, or NLP for short, is a branch of artificial intelligence (AI) that enables machines to understand human language and incorporate it into all kinds of processes.
Some well-known applications for NLP include:
(a) Text classification and sorting
The technique focuses on classifying texts into different categories or sorting a list of texts based on relevancy.
(b) Sentiment analysis
Sentiment analysis, known as opinion mining or emotion AI, is widely applied to voice of customer materials for applications that range from marketing to customer service to clinical medicine.
(c) Information extraction
Information extraction (IE) is the task of automatically extracting structured information from unstructured or semi-structured textual sources.
(d) Named-entity recognition
Named-entity recognition (NER) is actually a subtask of information extraction that seeks to locate and classify named entities mentioned in unstructured text into pre-defined categories.
(e) Speech recognition
Speech recognition, also known as automatic speech recognition (ASR), computer speech recognition, or speech-to-text, is a capability that enables a program to process human speech into a written format.
(f) Natural language understanding and generation (NLU & NLG)
There are altogether three natural language processing concepts and by now we have mentioned them all. At a high level, NLU and NLG are just components of NLP. Given how they intersect, they are commonly confused within the conversation.
(g) Machine translation
Machine translation is the process of using artificial intelligence (AI) to automatically translate content from a source language to a target language without any human input.
2. Database
In machine learning, a common task is the study and construction of algorithms that can learn from and make predictions on data. Therefore, the database is an essential component of machine learning. Three data sets are commonly used in different stages of the creation of a machine learning model: training, validation, and test sets.
Training data set: The training data is a set of examples used to fit the parameters of the ML model. Through training, the model will be able to recognize the important features of the data set.
Validation data set: The validation data set is used for trimming models’ coefficients, and comparing models to pick out the optimal one. The validation data set is different from the training data set, and cannot be used in the training section. Otherwise, overfitting may occur and adversely affect new data generation.
Test data set: Once the model is confirmed, the test data set is used for testing the model’s performance in a new dataset.
The splits of the three data sets used to be 50/25/25; however, some models may need less tuning or the training dataset can actually be a combination of training and validation (cross-validation), hence the ratio of training/test can be 70/30.
3. Computer vision
Computer vision is an artificial intelligence field focusing on training computers to analyze and understand figure and video data and to react to what they “see”.
Challenges in computer vision include:
Image classification: Image classification is a computer vision task that teaches computers to recognize certain images. It is the process of categorizing and labeling groups of pixels or vectors within an image based on specific rules. There have been models that are trained to recognize particular objects that appeared in specific places.
Target detection: Target detection is to teach a model to detect a particular class from a series of predefined categories, and use rectangles to circle them out. A popular application of target detection is the face recognition system. The model can detect every predefined matter and highlight them.
Image segmentation: Image segmentation is the process of partitioning a digital image into multiple segments (sets of pixels, also known as superpixels). The goal of segmentation is to simplify and/or change the representation of an image into something that is more meaningful and easier to analyze.
Significance test: Once sample data has been gathered through an observational study or experiment, statistical inference allows analysts to assess evidence in favor of some claim about the population from which the sample has been drawn. The methods of inference used to support or reject claims based on sample data are known as tests of significance.
4. Supervised learning
Supervised learning is the machine learning task of inferring a function from labeled training data. A supervised learning algorithm analyzes the training data and produces an inferred function, which can be used for mapping new examples. It is used when we know the correct answers from past data but need to predict future outcomes. An optimal scenario will allow the algorithm to correctly determine the class labels for unseen instances. This requires the learning algorithm to be generalized from the training data to unseen situations in a “reasonable” way.
5. Unsupervised learning
Unsupervised machine learning is the machine learning task of inferring a function to describe hidden structure from “unlabeled” data (a classification or categorization is not included in the observations). Since the examples given to the learner are unlabeled, there is no evaluation of the accuracy of the structure that is output by the relevant algorithm—which is one way of distinguishing unsupervised learning from supervised learning and reinforcement learning. It is used where there is no distinct correct answer, but we want to discover something new from the data.
6. Reinforcement learning
Reinforcement learning is different from what we just discussed. It involves constant improvements towards a predefined goal. Much like the process of gaming with computers, its goal is to train computers to take action in an environment so as to maximize some kind of cumulative reward. A well-known example of this is Alpha Go, the first computer program to defeat a professional human Go, player. Recently, reinforcement learning has also been applied in real-time bidding.
7. Neural network
Neural networks are computing systems inspired by the biological neural networks that constitute animal brains. Just like in brains where many neurons interconnect and form networks, an artificial neural network (ANN) is constituted by many layers. Every layer is an assemblage of a series of neurons. An ANN can process data consecutively, which means only the first layer is connected with the inputs, along with the layers increasing, an ANN gets more complicated. When layers get greatly large, the model becomes a deep learning model. It’s hard to define an ANN with a certain number of layers. 10 years ago, ANNs with only 3 layers are deep enough, now usually need 20 layers.
NNs have many variants, the ones in common use are:
- Convolutional Neural Network– it made great breakthroughs in computer vision
- Recurrent neural network– created to process data with sequence features, such as text and stock prices.
- Fully connected network– it’s the easiest model used for processing static/tabular data.
8. Overfitting
Overfitting is “the production of an analysis that corresponds too closely or exactly to a particular set of data, and may therefore fail to fit additional data or predict future observations reliably”. In other words, when a model learns from insufficient data, the deviation would occur, which may adversely affect the model. The problem of overfitting is common and critical at the same time.
A model that is overfitted takes random noises as data input or even an important signal to fit in. It is so specific to the original data that trying to apply it to data collected in the future would result in problematic or erroneous outcomes and therefore less-than-optimal decisions. It will appear to have a higher accuracy when you apply it to the training data, when in fact it will underperform in production when given new data. This happens a lot in some complicated models such as neural networks or acceleration gradient models.
Wrap Up
Whether you’re a beginner or looking to refresh your knowledge, grasping these concepts is crucial for navigating and excelling in the world of machine learning. We hope this article has made these terms more approachable and sparked your interest in exploring Machine Learning. Keep learning and stay curious!